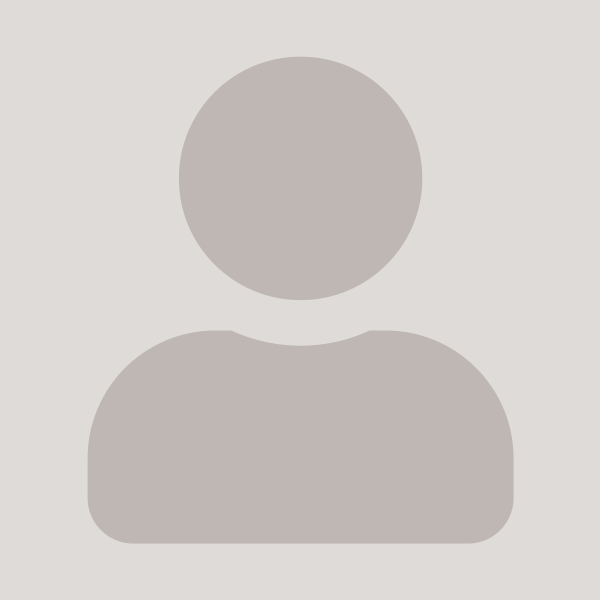
Malgorzata Bogdan
Professor
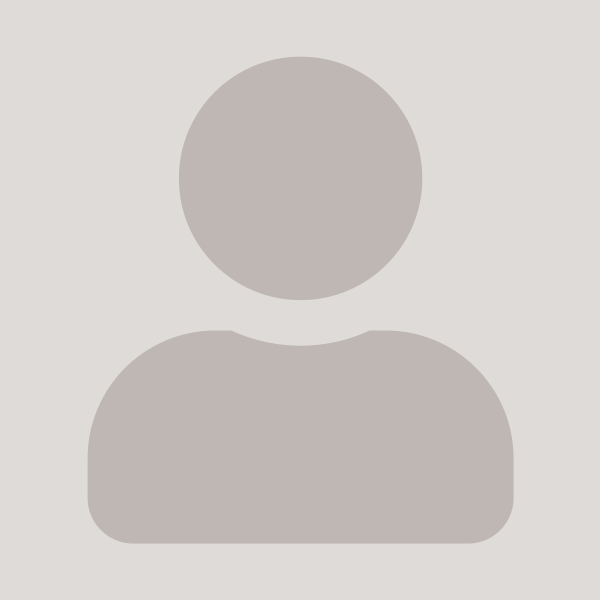
Rank-based lasso - Efficient methods for high-dimensional robust model selection
Författare
Summary, in English
We consider the problem of identifying significant predictors in large data bases, where the response variable depends on the linear combination of explanatory variables through an unknown monotonic link function, corrupted with the noise from the unknown distribution. We utilize the natural, robust and efficient approach, which relies on replacing values of the response variables by their ranks and then identifying significant predictors by using well known Lasso. We provide new consistency results for the proposed procedure (called,,RankLasso”) and extend the scope of its applications by proposing its thresholded and adaptive versions. Our theoretical results show that these modifications can identify the set of relevant predictors under a wide range of data generating scenarios. Theoretical results are supported by the simulation study and the real data analysis, which show that our methods can properly identify relevant predictors, even when the error terms come from the Cauchy distribution and the link function is nonlinear. They also demonstrate the superiority of the modified versions of RankLasso over its regular version in the case when predictors are substantially correlated. The numerical study shows also that RankLasso performs substantially better in model selection than LADLasso, which is a well established methodology for robust model selection.
Avdelning/ar
- Statistiska institutionen
Publiceringsår
2020-11
Språk
Engelska
Sidor
1-47
Publikation/Tidskrift/Serie
Journal of Machine Learning Research
Volym
21
Länkar
Dokumenttyp
Artikel i tidskrift
Förlag
Microtome Publishing
Ämne
- Probability Theory and Statistics
Nyckelord
- Lasso
- Model Selection
- Ranks
- Single Index Model
- Sparsity
- U-statistics
Status
Published
ISBN/ISSN/Övrigt
- ISSN: 1532-4435