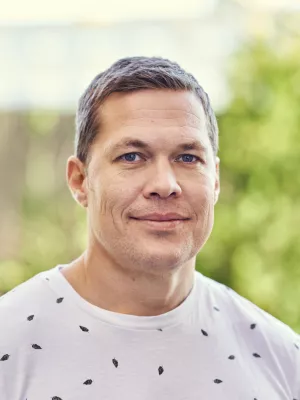
Jonas Wallin
Universitetslektor, Studierektor för forskarutbildningen, Statistiska institutionen
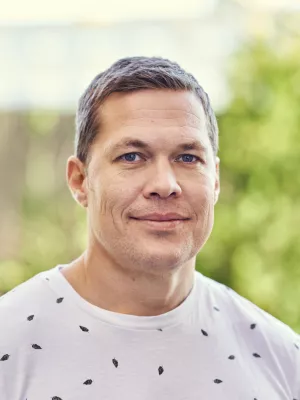
Efficient methods for Gaussian Markov random fields under sparse linear constraints
Författare
Summary, in English
Random Fields (GMRF) are computationally prohibitive when the number of
constraints is large. In some cases, such as for intrinsic GMRFs, they may even beunfeasible. We propose a new class of methods to overcome these challenges in the common case of sparse constraints, where one has a large number of constraints and each only involves a few elements. Our methods rely on a basis transformation into blocks of constrained versus non-constrained subspaces, and we show that the methods greatly outperform existing alternatives in terms of computational cost. By combining the proposed methods with the stochastic partial differential equation approach for Gaussian random fields, we also show how to formulate Gaussian process regression with linear constraints in a GMRF setting to reduce computational cost. This is illustrated in two applications with simulated data.
Avdelning/ar
- Statistiska institutionen
Publiceringsår
2021
Språk
Engelska
Publikation/Tidskrift/Serie
Advances in Neural Information Processing Systems
Volym
34
Länkar
Dokumenttyp
Konferensbidrag
Ämne
- Probability Theory and Statistics
Conference name
35th Conference on Neural Information Processing Systems (NeurIPS 2021)
Conference date
2021-12-06 - 2021-12-14
Status
Published
ISBN/ISSN/Övrigt
- ISBN: 9781713845393