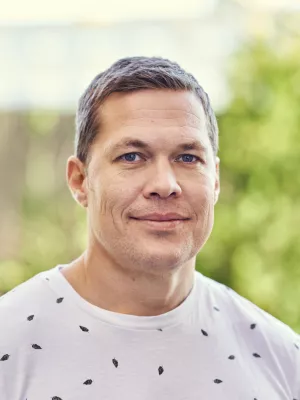
Jonas Wallin
Universitetslektor, Studierektor för forskarutbildningen, Statistiska institutionen
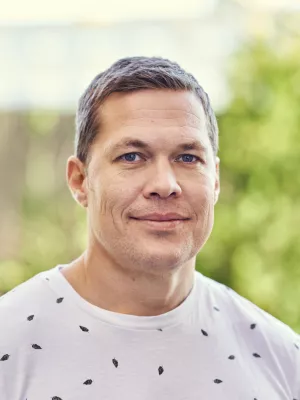
Efficient Adaptive MCMC Through Precision Estimation
Författare
Summary, in English
Avdelning/ar
- Statistiska institutionen
Publiceringsår
2018-10-02
Språk
Engelska
Sidor
887-897
Publikation/Tidskrift/Serie
Journal of Computational and Graphical Statistics
Volym
27
Issue
4
Dokumenttyp
Artikel i tidskrift
Förlag
American Statistical Association
Ämne
- Probability Theory and Statistics
Nyckelord
- AMCMC
- Gaussian Processes
Status
Published
ISBN/ISSN/Övrigt
- ISSN: 1537-2715