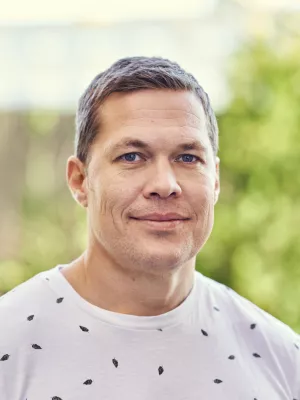
Jonas Wallin
Universitetslektor, Studierektor för forskarutbildningen, Statistiska institutionen
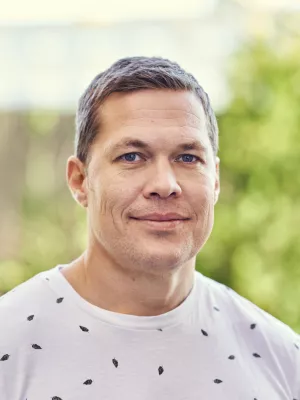
The Hessian Screening Rule
Författare
Redaktör
- S. Koyejo
- S. Mohamed
- A. Agarwal
- D. Belgrave
- K. Cho
- A. Oh
Summary, in English
Predictor screening rules, which discard predictors before fitting a model, have had considerable impact on the speed with which sparse regression problems, such as the lasso, can be solved. In this paper we present a new screening rule for solving the lasso path: the Hessian Screening Rule. The rule uses second-order information from the model to provide both effective screening, particularly in the case of high correlation, as well as accurate warm starts. The proposed rule outperforms all alternatives we study on simulated data sets with both low and high correlation for `1-regularized least-squares (the lasso) and logistic regression. It also performs best in general on the real data sets that we examine.
Avdelning/ar
- Statistiska institutionen
- Lunds universitet
Publiceringsår
2022-12-06
Språk
Engelska
Sidor
25404-25421
Publikation/Tidskrift/Serie
Advances in Neural Information Processing Systems
Volym
35
Dokumenttyp
Konferensbidrag
Förlag
Curran Associates, Inc
Ämne
- Probability Theory and Statistics
Conference name
36th Conference on Neural Information Processing Systems, NeurIPS 2022
Conference date
2022-11-28 - 2022-12-09
Conference place
New Orleans, United States
Status
Published
Projekt
- Optimization and Algorithms for Sparse Regression
ISBN/ISSN/Övrigt
- ISSN: 1049-5258
- ISBN: 9781713871088