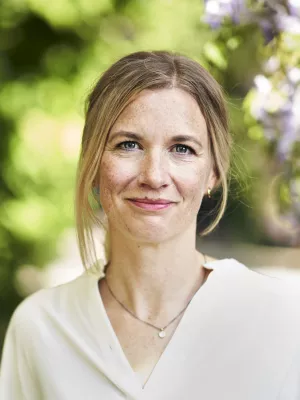
Kerstin Enflo
Professor
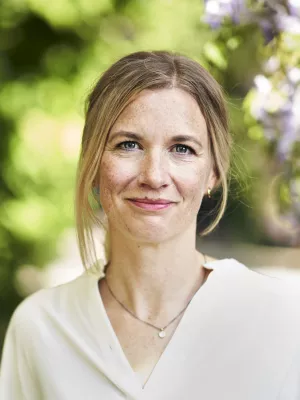
Reading the ransom: Methodological advancements in extracting the Swedish Wealth Tax of 1571
Författare
Summary, in English
We describe a deep learning method to read hand-written records from the 16th century. The method consists of a combination of a segmentation module and a Handwritten Text Recognition (HTR) module. The transformer-based HTR module exploits both language and image features in reading, classifying and extracting the position of each word on the page. The method is demonstrated on a unique historical document: The Swedish Wealth Tax of 1571. Results suggest that the segmentation module performs significantly better than the lay-out analysis implemented in state-of-the art programs, enabling us to trace many more text blocks correctly on each page. The HTR module has a low character error rate (CER), in addition to being able to classify words and help organize them into tabular formats. By demonstrating an automated process to transform loosely structured handwritten information from the 16th century into organized tables, our method should interest economic historians seeking to digitize and organize quantitative material from pre-industrial periods.
Avdelning/ar
- Ekonomisk-historiska institutionen
- Tillväxt, teknologisk förändring och ojämlikhet
- LTH profilområde: AI och digitalisering
- LTH profilområde: Teknik för hälsa
- eSSENCE: The e-Science Collaboration
- Matematisk statistik
- Biomedical Modelling and Computation
- Statistical Signal Processing Group
- Stroke Imaging Research group
- Matematik LTH
- ELLIIT: the Linköping-Lund initiative on IT and mobile communication
- Mathematical Imaging Group
Publiceringsår
2023
Språk
Engelska
Publikation/Tidskrift/Serie
Explorations in Economic History
Volym
87
Dokumenttyp
Artikel i tidskrift
Förlag
Elsevier
Ämne
- Economic History
Status
Published
Projekt
- Praise the people or praise the place: How culture and specialization drive long-term regional growth
Forskningsgrupp
- Biomedical Modelling and Computation
- Statistical Signal Processing Group
- Stroke Imaging Research group
- Mathematical Imaging Group
ISBN/ISSN/Övrigt
- ISSN: 0014-4983