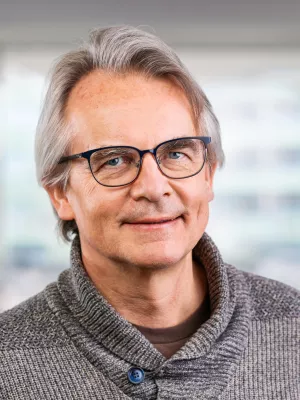
Krzysztof Podgórski
Professor, Prefekt Statistiska institutionen
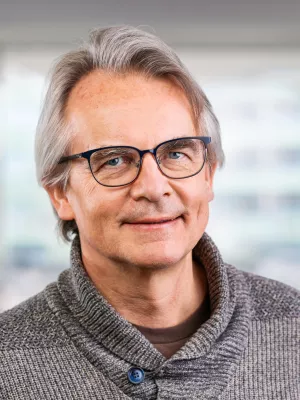
Empirically Driven Orthonormal Bases for Functional Data Analysis
Författare
Redaktör
- Fred J. Vermolen
- Cornelis Vuik
Summary, in English
In implementations of the functional data methods, the effect of the initial choice of an orthonormal basis has not been properly studied. Typically, several standard bases such as Fourier, wavelets, splines, etc. are considered to transform observed functional data and a choice is made without any formal criteria indicating which of the bases is preferable for the initial transformation of the data. In an attempt to address this issue, we propose a strictly data-driven method of orthonormal basis selection. The method uses B-splines and utilizes recently introduced efficient orthornormal bases called the splinets. The algorithm learns from the data in the machine learning style to efficiently place knots. The optimality criterion is based on the average (per functional data point) mean square error and is utilized both in the learning algorithms and in comparison studies. The latter indicate efficiency that could be used to analyze responses to a complex physical system.
Avdelning/ar
- Statistiska institutionen
Publiceringsår
2021
Språk
Engelska
Sidor
773-783
Publikation/Tidskrift/Serie
Lecture Notes in Computational Science and Engineering
Volym
139
Dokumenttyp
Konferensbidrag
Förlag
Springer Science and Business Media B.V.
Ämne
- Control Engineering
Conference name
European Conference on Numerical Mathematics and Advanced Applications, ENUMATH 2019
Conference date
2019-09-30 - 2019-10-04
Conference place
Egmond aan Zee, Netherlands
Status
Published
ISBN/ISSN/Övrigt
- ISSN: 1439-7358
- ISSN: 2197-7100
- ISBN: 9783030558734
- ISBN: 978-3-030-55874-1