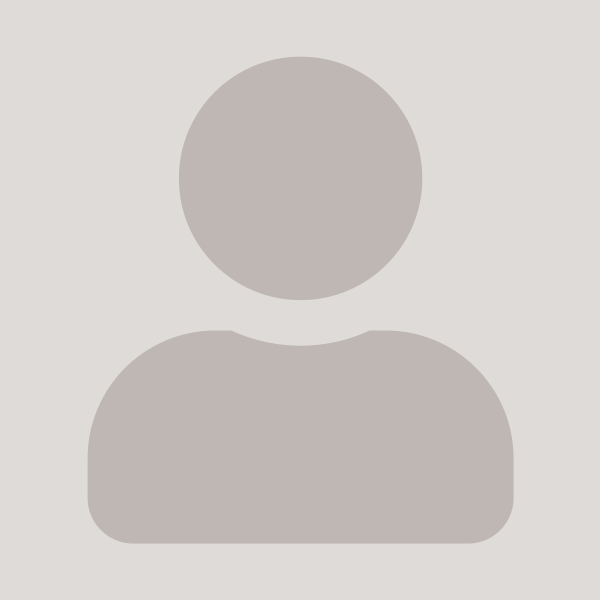
Malgorzata Bogdan
Professor
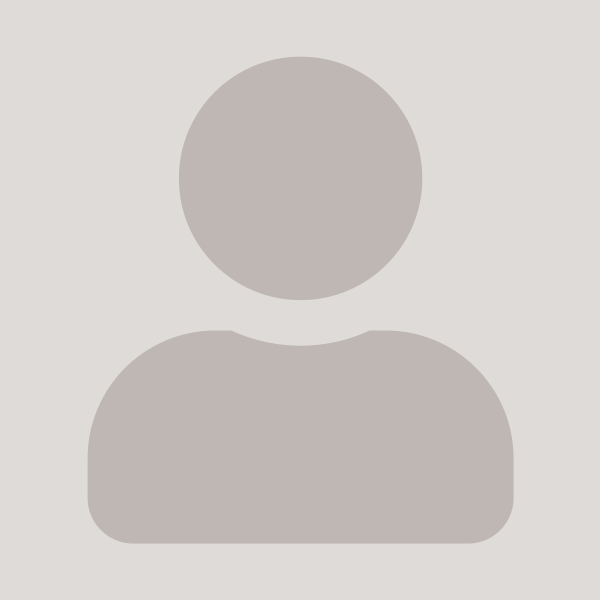
Inferring the Redshift of More than 150 GRBs with a Machine-learning Ensemble Model
Författare
Summary, in English
Gamma-ray bursts (GRBs), due to their high luminosities, are detected up to a redshift of 10, and thus have the potential to be vital cosmological probes of early processes in the Universe. Fulfilling this potential requires a large sample of GRBs with known redshifts, but due to observational limitations, only 11% have known redshifts (z). There have been numerous attempts to estimate redshifts via correlation studies, most of which have led to inaccurate predictions. To overcome this, we estimated GRB redshift via an ensemble-supervised machine-learning (ML) model that uses X-ray afterglows of long-duration GRBs observed by the Neil Gehrels Swift Observatory. The estimated redshifts are strongly correlated (a Pearson coefficient of 0.93) and have an rms error, namely, the square root of the average squared error <Δz2>, of 0.46 with the observed redshifts showing the reliability of this method. The addition of GRB afterglow parameters improves the predictions considerably by 63% compared to previous results in peer-reviewed literature. Finally, we use our ML model to infer the redshifts of 154 GRBs, which increase the known redshifts of long GRBs with plateaus by 94%, a significant milestone for enhancing GRB population studies that require large samples with redshift.
Avdelning/ar
- Statistiska institutionen
Publiceringsår
2024-03-01
Språk
Engelska
Publikation/Tidskrift/Serie
Astrophysical Journal, Supplement Series
Volym
271
Issue
1
Dokumenttyp
Artikel i tidskrift
Förlag
IOP Publishing
Ämne
- Probability Theory and Statistics
Status
Published
ISBN/ISSN/Övrigt
- ISSN: 0067-0049