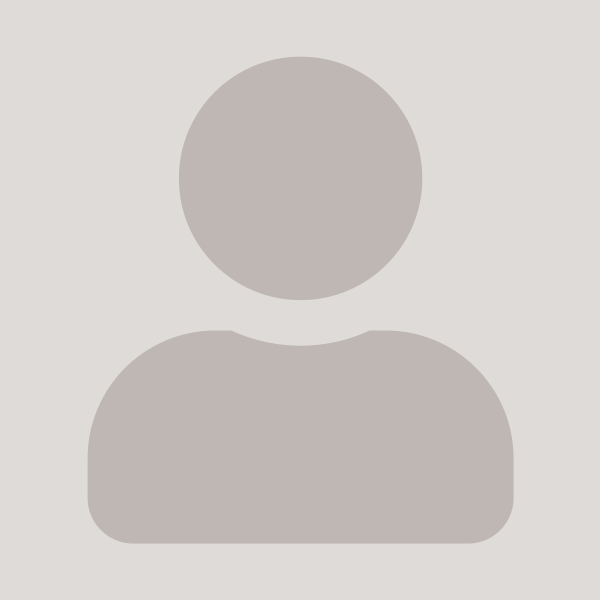
Malgorzata Bogdan
Professor
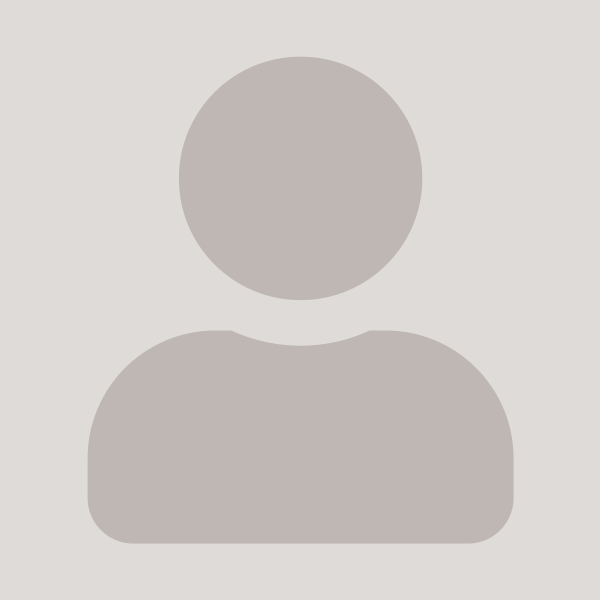
On the Asymptotic Properties of SLOPE
Författare
Summary, in English
Sorted L-One Penalized Estimator (SLOPE) is a relatively new convex optimization procedure for selecting predictors in high dimensional regression analyses. SLOPE extends LASSO by replacing the L1 penalty norm with a Sorted L1 norm, based on the non-increasing sequence of tuning parameters. This allows SLOPE to adapt to unknown sparsity and achieve an asymptotic minimax convergency rate under a wide range of high dimensional generalized linear models. Additionally, in the case when the design matrix is orthogonal, SLOPE with the sequence of tuning parameters λBH corresponding to the sequence of decaying thresholds for the Benjamini-Hochberg multiple testing correction provably controls the False Discovery Rate (FDR) in the multiple regression model. In this article we provide new asymptotic results on the properties of SLOPE when the elements of the design matrix are iid random variables from the Gaussian distribution. Specifically, we provide conditions under which the asymptotic FDR of SLOPE based on the sequence λBH converges to zero and the power converges to 1. We illustrate our theoretical asymptotic results with an extensive simulation study. We also provide precise formulas describing FDR of SLOPE under different loss functions, which sets the stage for future investigation on the model selection properties of SLOPE and its extensions.
Avdelning/ar
- Statistiska institutionen
Publiceringsår
2020
Språk
Engelska
Sidor
499-532
Publikation/Tidskrift/Serie
Sankhya A
Volym
82
Issue
2
Dokumenttyp
Artikel i tidskrift
Förlag
Springer
Ämne
- Probability Theory and Statistics
Nyckelord
- 62J05
- Convex optimization
- High dimensional regression
- Model selection
- Multiple testing
- Primary; 62J07
- Secondary 62F12
Status
Published
ISBN/ISSN/Övrigt
- ISSN: 0976-836X